We start by meeting to review your project and what data you have already generated. We will determine what stage you’re at and what additional steps are necessary to get you where you want to go.
We will perform a careful review of the academic and patent literature around your target, and curate and add relevant data from the literature to increase the size of our dataset. This can improve the predictive ability of models we build. Any data we discover will be discussed with you to ensure its validity and appropriateness for incorporation into models. We will carefully standardize all data by neutralizing compounds, finding preferred tautomers, removing metal ions and salts, and removing duplicates.
We will then use our AI algorithms to construct QSAR models based on your data, that which we find from literature, and our novel molecular/atomic descriptors. Conversely, we can incorporate any QSAR/QSPR models that you may have generated internally.
Prior to performing the first AIDD run, we’ll put on our medicinal chemist hats and closely inspect the data to better guide the AIDD process. This includes utilizing best-in-class cheminformatic toolkits, identifying key activity cliffs via matched molecular pair analysis (MMPA), generating classes and scaffolds for each class, then generating and analyzing R-tables to provide clues for which parts of the molecule may be the best to evolve.
We will then perform our first AIDD run with parameters for optimization decided upon collaboratively.
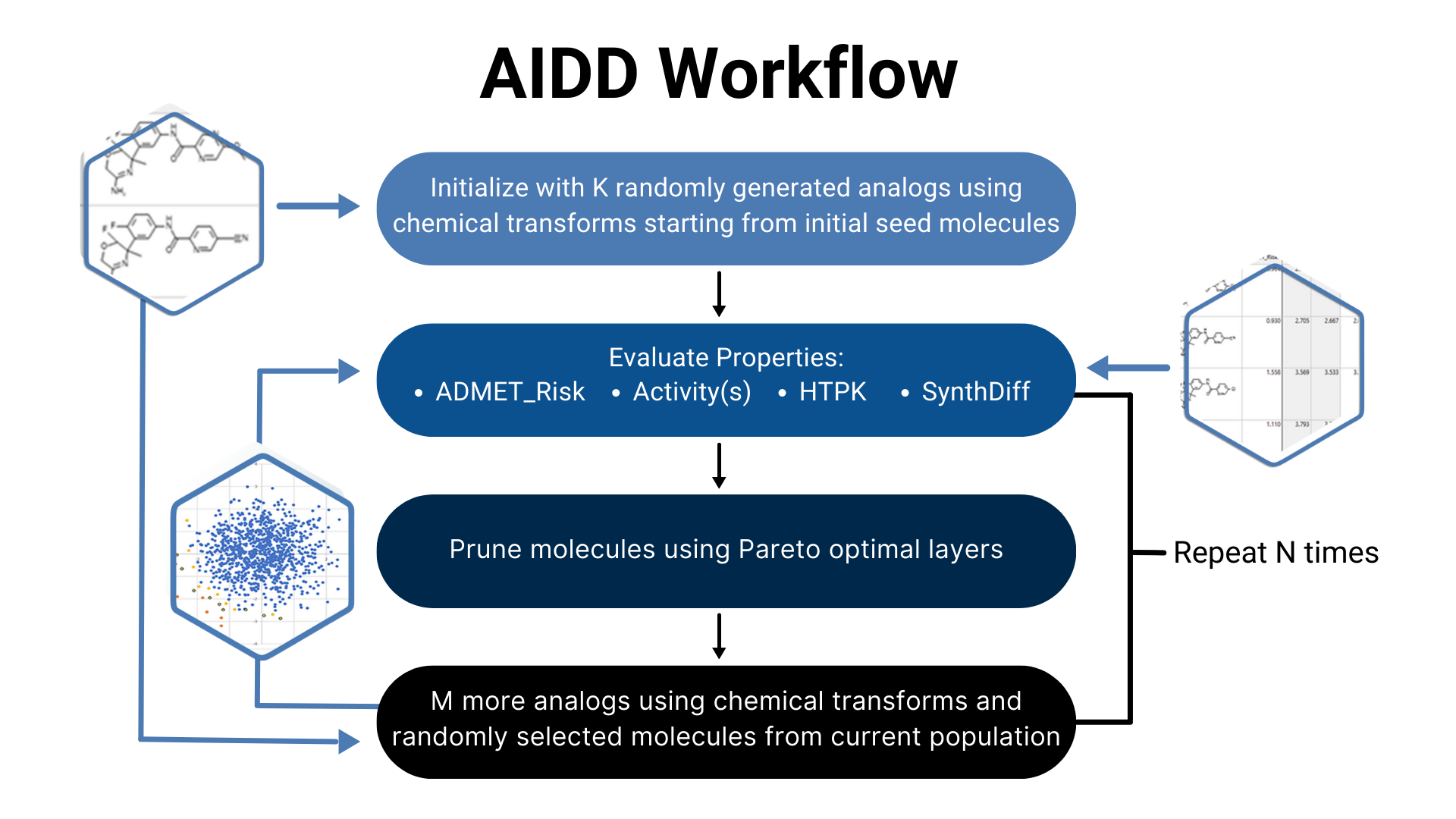
We typically optimize 4-5 parameters simultaneously, the most common parameters being target activity, ADMET Risk™, preclinical/clinical PK endpoints (e.g., bioavailability) and synthetic difficulty. However, there are over 100 parameters for which your molecules can be optimized.
Our experts will then filter results for novelty (by similarity searches against patent databases) and commercial availability (by searching chemical manufacturer databases such as Enamine REAL). If these searches limit the potential number of molecules, a second round of AIDD will be performed.